
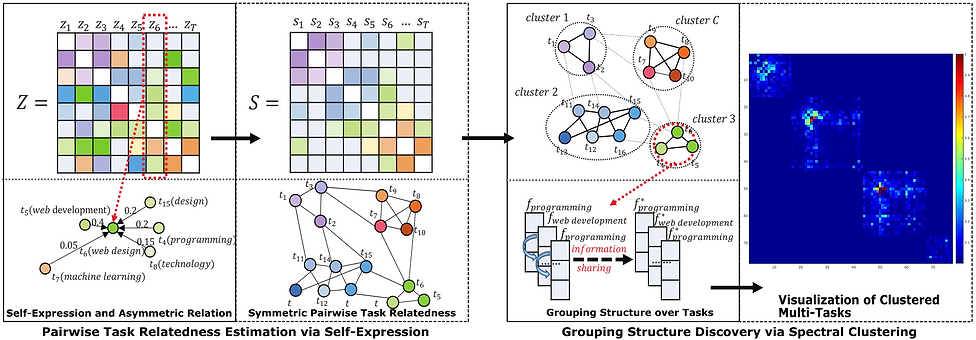
Jointly learning multiple relevant tasks has theoretically and empirically shown efficacy in transferring knowledge and boosting substantial performance improvement. Most of existing multi-task learning methods assume that all the tasks are either related in a uniform way or following a certain pre-defined structure, such as tree, graph or clusters. To uncover task relatedness, most of the existing efforts focus on constructing task relatedness at the feature-level. However, feature-level task relatedness may not be robust due to insufficient samples or outlier tasks, and hence lead to negative transfer among trivial tasks. Toward this end, we propose a novel fundamental self-expressed clustered multi-task learning model, which is capable of automatically uncovering more accurate task relatedness from the task-level, exploiting grouping structure over tasks, and hence jointly reinforcing the prediction performance. In particular, distinct from existing clustered multi-task learning methods, we involve the following factors into our proposed model: (1) self-expressed representation of relevant tasks to learn task relatedness from task-level, where each task can be represented as an affine combination of its relevant tasks; and (2) grouping structure on the resultant relatedness to encourage the compatible knowledge within the same groups. Moreover, we theoretically derived the solution of our method and applied it to a real-world scenario, i.e., user interest inference from social media (e.g., Facebook, Twitter, and Quora), and verified the inference results regarding multiple metrics from different aspects. Extensive experiments have demonstrated the superiority of our model over several state-of-the-art methods.